Without data, you’re just another person with an opinion

Usually, big unstructured data has specific characteristics that we have difficulty understanding.
Usually, big unstructured data has specific characteristics that we have difficulty understanding. Therefore it is our nature to shape this data into a form people can naturally process.
We all use software to capture, digitise, handle and integrate vast amounts of data within a company. This process is often driven by short-term business value, such as capturing intellectual property or meeting regulatory compliance requirements. However, for a longer-term digital transformation to drive change within a company, we need to cleanse, manage and store it as organised, structured data.
The organised data now provides a company with a substantial chance to analyse and reveal changes that may be made to drive efficiencies across the company and deliver the value extracted from the data.
Why is data necessary for my business’ future?
Data analysis helps you understand how you got to where you are today. Moreover, it lets you to know how to get to where you want to be tomorrow.
Data allows you to:
- understand where you can improve
- pinpoint your weak spots
- manage risks associated with certain activities
Having access to statistically valid data helps companies know the right questions to ask and gives them a framework for deciding what’s important. But how can companies validate the integrity of the data they are working with and feel confident that it forms a good platform when driving critical changes within the business?
Enter, statistics
The field of statistics is concerned with collecting, analysing, interpreting, and presenting data.
Statistics is the field that can help us understand how to use this data to:
- gain a better understanding of the world around us
- make decisions
- help make predictions
Many statistical tests make assumptions about the underlying data under study. Say you’re reading the survey results or even performing your analysis. It’s essential to understand what assumptions need to be made for the results to be reliable (e.g., does this information include outliers or have they been removed).
Descriptive statistics such as summaries, charts, and tables can help us better understand existing data. For example, if we want to calculate the average cost of an item, visualise the cost distribution depending on the region, or identify the frequency of an event, descriptive statistics can help. Using descriptive statistics, we can understand the cost information much quicker than just staring at the raw data.
However, we must be aware of the caveats
Unfortunately, charts can often be misleading if you don’t understand the underlying data. They can sometimes oversimplify complex information, or even introduce bias. An awareness of this bias can help you avoid misreading these specific charts.
Another aspect of data that needs to be understood is the relationship between confounding variables (e.g., missing location data). These variables are unaccounted for and can confound the results, leading to unreliable findings.
Multicollinearity is studied in data science and is becoming a critical tool in making data-based decisions. Understanding that the variables used for your analysis are independent is essential. Due to multicollinearity, we may get different coefficients (regression coefficients are the quantities by which the variables in a regression equation are multiplied) for the same factors, leading to wrong interpretations, which could have profound effects on your results.
Another question when studying data is how likely an event will happen. A basic understanding of the probability of an event happening can also help you make informed decisions in the real world.
Statistically, valid regression models can help companies dig deep into their data mine and unearth valuable information.
Now, let’s talk about regression models and estimating
Regression models (e.g., linear regression) make predictions about future phenomena. Each model allows you to make predictions of some variable based on the value of another variable (e.g., cost vs quantity). Regression models are often presented in chart form. However, this chart only represents the regression model equation.
Companies can use regression models when doing top-down estimates. Top-down estimating is practical for the initial stage of strategic decision-making. Additionally, it is used when the information required to develop accurate duration and cost estimates is unavailable in the project’s initial phase. This is where using regression models to help predict a project cost can be convenient as the underlying data used to create the model had been built with statistically validated data and can provide reliable predictive project costs.
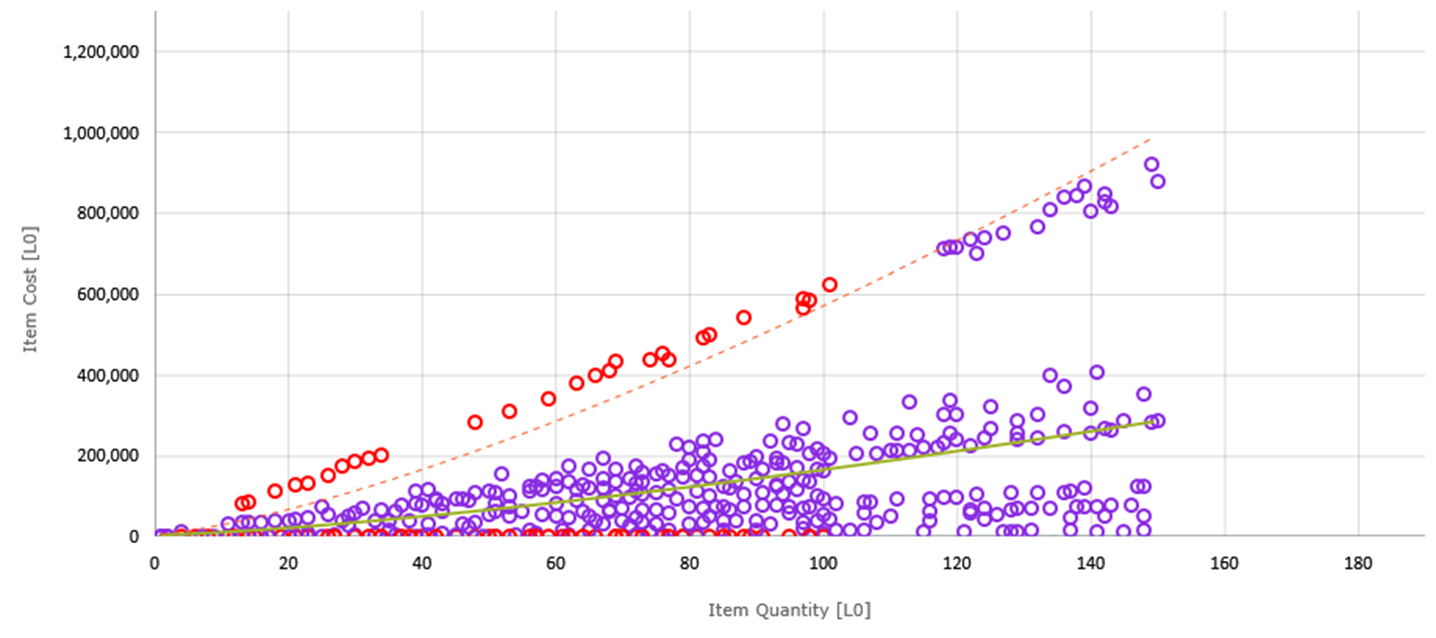
The benefits of regression models
Using regression models, companies can quickly create ballpark project costs of different options and then use first principles to detail a feasible option.
Regression models can predict the asset value of company infrastructure installed around the country. Asset valuations often form part of the company’s annual letter to shareholders or for specific government regulatory requirements.
Regression models can:
- predict the cost of items that form part of a project without requiring supplier quotes
- predict the cost of all estimated projects compared to actual project costs and identify irregularities
- help fine-tune project costs and increase cost efficiency within your business without compromising quality
- aid in determining the outliers in your cost estimates and minimise risks associated with different interpretations of estimating data
- assess the effect of inflation within a project when forecasting a future cost with or without inflation impact applied
- perform regionalised cost analysis for a specific project item
Furthermore, as we capture all the underlying information as part of generating a regression model, it is fully transparent to satisfy regulatory and auditability requirements.
So, how do regression models work?
Building a regression model requires multiple phases. We first must capture and cleanse the data. The analysis applied to this data is then validated by a set of statistics that guide the cost modeller when choosing the correct information to use as part of the data set that will be used for a regression model.
Once we generate a model, we must manage the entire life cycle. By this, we mean developing, updating, and archiving. When you are accessing years of estimated project data for analysis that does not require any re-keying of data when using the statistical application, Benchmark Estimating Analytics (BEA), it can unlock the intellectual property already contained within the data. This helps drive cost efficiencies within your business and future projects while providing your company with new insights.
We’re here to help make all this easy
Benchmark’s Data and Intelligence team can assist companies in data management. We help companies with information migration and management and access software tools to help develop project cost estimates using bottom-up and top-down methods. We can build dashboard summary views of critical intelligence in your business while maintaining data integrity.
It is our assumptions that give voice to the data, and the quality of these assumptions dramatically influences the quality of insights.
Much like William Edwards Deming (engineer, statistician, professor) quotes, “Without data, you’re just another person with an opinion.”